- The Inference Times
- Posts
- Godfather of AI quits Google to warn us: Aliens are here!
Godfather of AI quits Google to warn us: Aliens are here!
Plus: The biggest news since ChatGPT, a big leak from Google, the end of education and more
Welcome to the seventh issue of The Inference Times! Thanks to your feedback we’re going to shoot for 1-2 issues a week!
Today we hear about aliens walking among us, dive into a big leak from Google, cover the biggest advance in AI since ChatGPT, learn about the end of education as we know it and much, much more!
Like what you see? Please forward this to anyone you know who might enjoy it!
📰 The Inference Times: Front Page
1) Godfather of AI quits Google to warn us all: Aliens are here and we just didn’t notice because they speak great English

Geoffrey Hinton, who along with Yann Lecun (head of Meta AI research), won the prestigious Turing award for his contributions to AI, decided to quit his gig at Google. His reasons are varied, but center around gaining flexibility to express his deep concerns for the risk presented by AI.
The most striking quote: “These things (large language models) are totally different from us. Sometimes I think it’s as if aliens had landed and people haven’t realized because they speak very good English.”

Geoffrey Hinton’s rendition of a language model alien that speaks lovely English
Folks near to developments in generative AI may be familiar with the slightly breathless warnings of Eliezer Yudkowski. In contrast, Geoffrey Hinton is highly credible: He was responsible for the earliest neural networks, discovered key optimization techniques like back-propagation and more. Heck, he was even the PhD adviser for one of OpenAI’s founders.
While Yann LeCun is spending his time downplaying the potential of large language models, Hinton highlights their remarkable strengths:
“It’s a completely different form of intelligence: A new and better form of intelligence… It’s scary when you see that, it’s a sudden flip.”
“Our brains have 100 trillion connections, large language models have up to half a trillion, a trillion at most. Yet GPT-4 knows hundreds of times more than any one person does. So maybe it’s actually got a much better learning algorithm than us.”
The traditional criticism of machine learning is it requires vastly more samples to learn than a human: It’s rate of learning is extremely slow.
Hinton disagrees here as well: “The bottom falls out of that argument as soon as you take one of these large language models and train it to do something new. It can learn new tasks extremely quickly.”
As for solutions, Hinton intends to work with industry leaders and politicians on regulations and takes inspiration on international norms against use of chemical weapons.
2) Google: We have no moat, but neither does OpenAI
This is a fascinating memo that everyone with an interest in generative AI should read in full.
First, it makes a convincing argument that Google (and OpenAI) will not be able to keep pace with open models.
Second, it is the single best overview of the progress of open language models yet written.
Some remarkable quotes: “The barrier to entry for training and experimentation has dropped from the total output of a major research organization to one person, an evening, and a beefy laptop.”

Tiny open models have come to rival Google’s massive, costly Bard agent in roughly 3 weeks!
“[open models] are doing things with $100 and 13B params that we struggle with at $10M and 540B”
Initially, my assumption was that we would have several closed-source large models trained by major organization like Google, Facebook and OpenAI.
The article makes a convincing case that smaller models iterated at a greater pace will beat large models. And the latter are and will be more of a liability than an asset.
Read it in full.
3) ChatGPT drops code interpreter: Video editor, data scientist and more
OpenAI has a closed beta of ChatGPT that gives their chat agent access to a code interpreter. The results are impressive! We played with two examples…
Video Editor
Upload a video file and ask it to stretch it, transcode it and apply a zoom effect? Done, done and done.
Basic video editing in ChatGPT, converting uploaded GIF to longer MP4 with slow zoom: twitter.com/i/web/status/1…
— Riley Goodside (@goodside)
5:09 AM • Apr 30, 2023
Data Scientist
Give it a CSV or Excel file and it will answer questions like: What were our company’s top-selling regions? What areas saw the highest crime rates?
But it goes several steps further: Can you run some regressions to look for patterns in the data? Can you perform diagnostics to check the validity of these relationships? It even catches missing values and removes them or imputes their values.

The code generated to answer the questions can be reviewed and audited. Wharton professor Ethan Mollick’s article has many more examples.
It’s an open secret that many data science roles consist of answering relatively basic business analytics questions.
These capabilities will increase self-service abilities for business roles even as they give the most capable business analysts dramatically more leverage.
In the future, we’ll spend very little time entering formulas in spreadsheet cells and more time asking questions of datasets.
And more
Because GPT-4 has access to the Python programming language and libraries, there’s no limit to what it can do!
The model itself is unable to describe all the potential inputs and outputs!
Find all the lighthouses that are within 10 miles of a Frank Lloyd Wright house? Yep. Analyze free-to-paid conversion funnel? That too. Download a photo of your face and draw a box around it? Uhuh. Create an entire lecture and synthesize human voice to deliver it? We’re there.
Feels like the biggest development since GPT-4 or ChatGPT.
4) Education is shifting under our feet
Chegg’s stock plummeted, losing 50% of its value on news that AI tools like ChatGPT are reducing paid subscriber growth.
Students turning not to study materials, but rather cribbing answers from an AI chatbot would seem to be dystopian future for education.
Fortunately, the real story is more nuanced - students are trading one (paid) solution to download quiz and homework answers for a (free) chatbot. Homeostasis in cheating is maintained even as we witness disruption from below.
While ChatGPT (mis)use is booming, Sal Khan, founder of Khan Academy presents a rosier vision for education in a recent TED talk.

The presentation is inspiring: Live examples show the tutor helping students through a variety of subjects, from math, to literature, writing and computer science. 1:1 AI tutors will increase education outcomes even as they narrow achievement gaps

5) AI is already shaking up professional services
Legal AI startup Harvey.ai has signed a $21M deal with Sequoia just 6 months after its $5M raise from OpenAI’s startup fund. The deal did not carry a public valuation, but early rumors of the deal placed the valuation at $150M post-money.
Despite a waiting list 15,000 firms deep, Harvey has been taking it slow. Reporting as of early April had their client base at just 2 customers. That includes: international law firm Allen & Overy, who purportedly bought $1 million in usage tokens for 3,500 lawyers across 43 offices. The second customer is PriceWaterHouseCooper, which enjoys exclusive access among the big 4 consultancies.
PriceWaterHouseCoopers separately announced a $1 billion investment into AI. Meanwhile, IBM has paused hiring while it analyzes the impact of generative AI on consulting practice and even suggested they were contemplating replacing nearly 8,000 jobs with AI.
There is abundant precedence for technology transforming professional services. Computer indexing of documents and keyword search transformed legal tasks like doc review — hundreds of paralegals are no longer employed to read through 1,000s of pages of documents for keywords in legal discovery. Doc review in major cases went from $2 million in 1978 to $100k 2011.
Now, technology will move up the ladder of sophistication, replacing the work of 1st year law and consulting associates: Lit review, slide creation, financial modeling and more. A recent article makes a strong case that it is highly-educated, highly-paid, white-collar occupations that will be most impacted by AI.
But the impact on overall employment in these fields is ambiguous. There are more lawyers today than 10 years ago, for instance. Legal service demand may increase as it becomes cheaper, but laws may also become more complex as they get easier to parse while lawsuits become more common as they become cheaper to wage.

🌎 Around the web
The medical AI future is already upon us
OpenAI’s solution is already being used to read and respond to patient messages, albeit with a (human) doctor in the loop.
The messaging pilot is taking place at UC San Diego, UW Health and Stanford Health. As soon as a doctor clicks on a patient message, the instantly generates a draft message that mentions their recent visit, current medication and more.
WSJ, without paywall.Bing chat to get plugins too
Microsoft Bing’s chat tool will soon have plugins as well. If you haven’t gotten access to ChatGPT plugins, Bing may soon have you covered.
China proposes strict rules for generative AI
Geoffrey Hinton didn’t have much faith in Western governments coordinating on AI regulation, but it seems like China is on it!In addition to avoiding discriminatory, violent, obscene, false, or harmful content, generative AI must respect social virtue, good public customs and the Socialist Core Values. There are a host of other proposed principles that generative AI must adhere to, from data privacy and transparency to avoiding user addiction to the services.
Biden and Kamala talk AI
They’re promoting responsible AI innovation, including an AI Bill of Rights. No, not rights for the AI — this is rights for humans in the era of generative AI.
Companies ban generative AI
Samsung banned employees use of ChatGPT after the bug that exposed chat histories to other users. Meanwhile other companies have banned or restricted its use: Bank of America, Citigroup, Goldman Sachs, Wells Fargo and more.
It’s pretty clear we’ll see demand for ‘on-prem’ large language models so companies don’t need to trust their data to outside services. That means more demand for open source self-hosted models, alternate hosting paradigms for closed models or a combination of both.
Amazon plans LLM-superpowered Alexa
A bit of a no-duh announcement, but it’s further evidence for the degree to which OpenAI caught the tech majors napping.
🇮🇹 ChatGPT returns to Italy
Looks like OpenAI’s privacy concessions to EU regulators were enough to restore access to Italy.
Stability.ai launches StableVicuna
Instruction tuned Vicuna, hosted on HuggingFace.
🔧 Tool Time:
New AI coding assistants
The two new entries in the coding assistant space: StarCoder from HuggingFace and ServiceNow (announcement, demo, repo) and Replit’s latest entry (announcement, demo, repo).
A practitioners guide to Llama and family
Curious how all the confusing Llama family of models relate to each other? Can’t tell Llama from Alpaca from Vicuna? This helpful writeup covers how all these various models relate to each other in size, capabilities, architecture and more.
AutoPR: Action to create a GitHub pull request
This tool implements a GitHub action that will autonomously write a pull request given an issue describing the desired code changes.
Multilingual speech synthesis
ElevenLabs Multilingual Speech Synthesis https://beta.elevenlabs.io
🧪 Research:
🪞 Neuron, neuron on the wall: Who is most critical of them all?
Ever wondered what the neurons in a neural net actually… do? This study seeks to answer this question in the Pythia Large Language Model.
The researchers identify neurons that are highly activated for tokens (ie words) from various contexts: French, the Go programming language and patent filings. “Ablating” (removing) these neurons degrades language model performance in the domain, but be relatively unaffected in others.
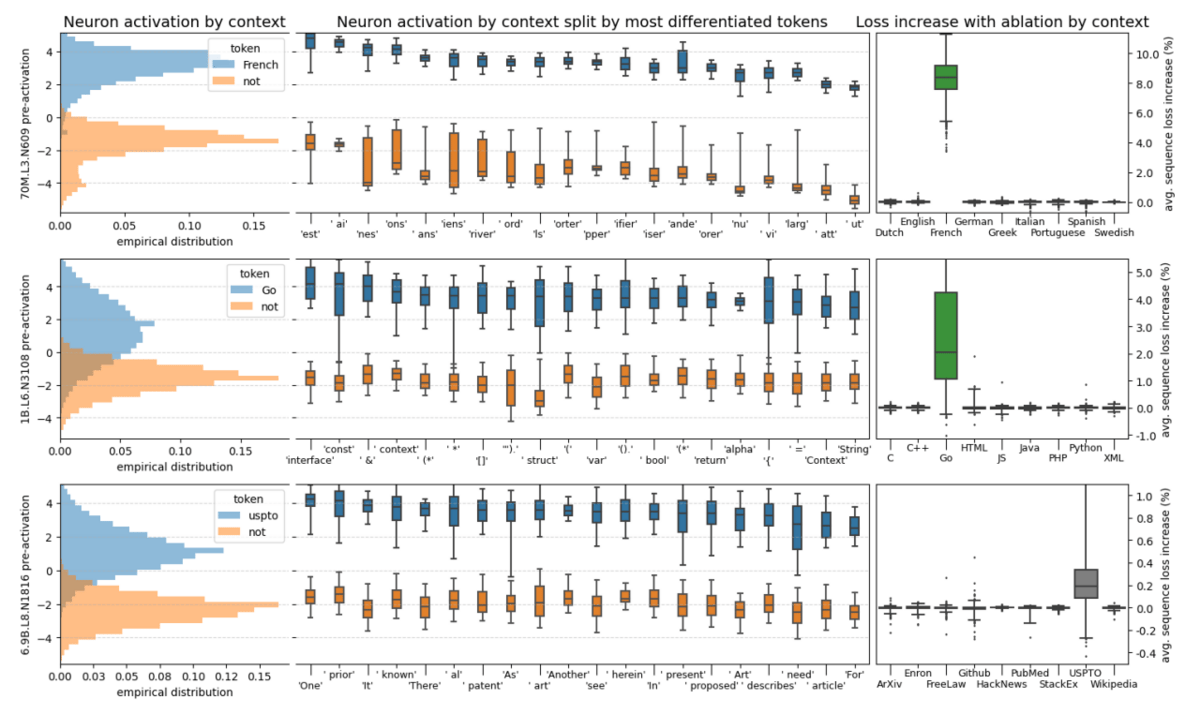
Specific neurons activate for tokens from one context (blue, on left left). Removing these neurons worsens performance in that domain, but not others (loss increases, on right)
The researchers also identify combinations of features that represent higher-order concepts, for instance living female soccer players, end-of-sentence neurons and neurons that are highly activated for player names and words associated with particular sports.

A neuron that fires for hockey players (left) also fires for hockey-related words (right).
🗜️ Under pressure: To Compress or Not To Compress
That is the question Meta AI labs director Yann LeCun contemplates in a recent preprint with collaborator Ravid Schwatz-Ziv. The article presents an interesting information-theoretic analysis of unsupervised vs self-supervised learning and the tradeoff between rote memorization of data (useful for important text) and compression of data (critical to achieve learning)
👩💼 Jobs at risk: White collar, highly-educated, highly-paid
An analysis of jobs most at risk of disruption by AI.

💡⚡️ AI Wisdom
